A Minimum Variance Spectral Estimation-Based Time-Frequency Analysis for Click-Evoked Otoacoustic Emissions
Z. G. Zhang 1,3 , V. W. Zhang 2, S. C. Chan 3, B. McPherson2, Y. Hu 1
1 Department of Orthopaedics and Traumatology The University of Hong Kong, Pokfulam Road, Hong Kong
2 Division of Speech and Hearing Sciences The University of Hong Kong, Pokfulam Road, Hong Kong
3 Department of Electrical & Electronic Engineering, The University of Hong Kong, Pokfulam Road, Hong Kong
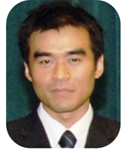 |
Dr Z. G. Zhang received his B.Sc degree from Tianjin University, China, in 2000, and graduated with an M.Phil degree in electronic engineering from the University of Science and Technology of China in 2003. He obtained his Ph.D. degree in the Department of Electrical and Electronic Engineering at the University of Hong Kong in 2008. He was a research assistant in Department of Orthopaedics and Traumatology, the University of Hong Kong, during 2008. He is currently a postdoctoral fellow in Department of Electrical and Electronic Engineering at the University of Hong Kong. His research interests are in general area of digital and biomedical signal processing. |
1. Introduction
Traditionally, CEOAE responses are analyzed in the frequency domain via the Fourier transform. This is very useful for a primary description of CEOAE responses and is a method which aligns with clinical needs. However, CEOAEs are nonstationary signals with frequency dispersion along the time axis. Therefore, traditional spectral analysis using Fourier methods may miss some important temporal information. Recently, time-frequency analysis (TFA) of OAE has attracted increasing interest. Various TFA techniques, such as the Wigner-Ville distribution (WVD) [1], the STFT [2], the wavelet transform [3], and the matching pursuit [4], have been applied systematically to study the characteristics of different types of OAE signals. The WVD has high time-frequency resolution but it often results in severe cross-terms. The STFT originates from the Fourier transform and it faces a basic time-frequency resolution tradeoff problem, as it cannot achieve good time resolution and frequency resolution simultaneously. Wavelet transform has been proved to an effective technique for CEOAE analysis, but it has a degraded frequency resolution for high-frequency components and a degraded time resolution for low-frequency components. Matching pursuit can overcome limitations in wavelet transform by using a greedy search to expand the signal into a set of basic atoms, but its computational complexity is too heavy.
In this study, a new nonparametric TFA method based on minimum variance spectral estimation (MVSE) is introduced. The MVSE is a spectral estimation algorithm which can provide higher resolution than other nonparametric spectral estimates such as the periodogram [5]. Using the same sliding window operation as in STFT, the conventional MVSE in the frequency domain can be extended to a windowed MVSE (WMVSE). Inspired by the window selection criterion of wavelet transform, we address the window selection problem of WMVSE by using a long window at low frequencies and using a short window for high frequencies. The frequency-dependent window size produces the frequency-dependent WMVSE (FDWMVSE), which can be used to analyze the time-frequency characteristics of CEOAEs.
2. Materials and Mthods
2.1. Experimental
protocols
A total of 100
neonatal CEOAE responses used in the experiments were recorded from well-baby
nurseries at the Hong Kong
Adventist Hospital. The average ambient
room noise level with OAE equip ment
in operation was less than 50 dBA. All the data
fulfilled the following CEOAE criteria: stimulus stability;
75%; whole wave reproducibility ³
70%; overall CEOAE response ³
5 dB SPL; at least three of five test frequency bands centered at 1, 1.4, 2, 2 .8 and 4 kHz with
SNR ³
3 dB. OAE data were
collected using ILO USB equipment plus V6 OAE clinical software (Otodynamics
Ltd., UK). Nonlinear “ QuickScreen ” mode was used for data
collection ( stimulus level of
75-80 dB peSPL ; analysis window of 12. 8ms ). Res ponse stopping
criteria for CEOAE measurements required at least 70 OAE stimuli presentations,
or, if no clear CEOAE response at 70 presentations, up to 260 responses were
obtained.
2.2. Frequency-dependent
windowed m inimum variance
spectrum estimation (FDWMVSE)
In
MVSE, an adaptive filter is used to minimize the energy of other frequency
components except for the desired frequency component, hence the name minimum
variance. By recording the signal energy of the adaptive filter designed for
different freq uencies,
an energy spectrum of the input signal is obtained. Suppose the OAE signal is
and the filter order is ,
the MVSE at a frequency of
interest
is calculated as
|
,
|
(1)
|
where
is the by
autocorrelation
matrix of
and .
To
track the time-varying frequency contents of nonstationary CEOAEs , a windowed MVSE (WMVSE) is proposed and its
idea is 1) to slide the center of
a -size
window
to each time instant ,
2) to window the input
signal
to yield -size
data segments ,
3) to calculate the autocorrelation matrix from ,
and 4) to obtain the WMVSE
at time instant as
|
.
|
(2)
|
The time-frequency
resolution tradeoff proble m
also exists in WMVSE, and the WMVSE with a
fixed
and
for different frequency bands is not a
good method . Motivated by the wavelet transfor m that uses adaptive
and ,
a new frequency-dependent
WMVSE (FDWMVSE) method is proposed (see reference [ 6 ] for full details) . The FDWMVSE uses
frequency-dependent windows: shorter windows for short-duration hi gh-frequency CEOAE
components to achieve good time resolution and large window sizes for
long-duration low-frequency components to obtain high frequency resolution. More precisely , in the FDWMVSE
method , the
frequency-dependent window size
is adjusted to be inverse proportional to frequency .
The relation can be approximately expressed using a
linear function as ,
where and
are parameters fo r varying the
window size function. In the study, with the help of a
synthetic CEOAE model [3] , we estimated these parameters
in a trial and error manner and determined the window size
function .
Then, the
frequency-dependent order
was chosen as .
3. Results
One real CEOAE sample is illustrated in
Fig. 1 to show the effectiveness of
the proposed FDWMVSE method . For comparison , the analysis
results of the wavelet transform (scalogram) method are also shown . In Fig. 1 , two components
located between 3.5 kHz and 4 kHz cannot be identified in the scalogram but they
are shown in the result of FDWMVSE. Based on the periodogram and their
positions, these two high-frequency components should not be spurious .
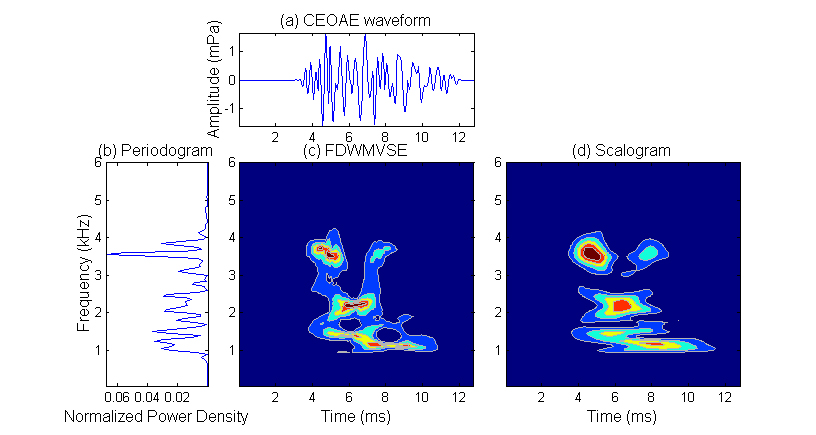
Fig. 1 . Time-frequency
representations
of one real CEOAE signal using FD WMVSE and scalogram .
The performance of
the proposed FDWMVSE method was further assessed and
compared with wavelet transform quantitatively by the parameters
of peaks det ected in the
time-frequency distributions. To study the characteristics of CEOAE
components in different frequency bands,
the whole frequency range was divided into six bands: 0 < f <= 1 kHz, 1 kHz < f <= 2 kHz, 2 kHz < f <= 3 kHz, 3 kHz < f <= 4 kHz, 4 kHz < f <= 5 kHz, and f > 5 kHz. In each frequency
band, the location of its peak was detected as the time-frequency
point with the maximum value of the time-frequency
distribution in the f requency
band . The latency and frequency of
peaks can reveal meaningful
information regarding CEOAE components.
Further, t o compare the
frequency resolution of various TFA method s , the peak width,
which denotes 80% energy density of the peak power in each
frequency
band , was measured in
both time and frequency dimensions. A narrow peak indicates a relatively high
resolution, whereas a wide peak means low resolution.
Based on the peak detection
approach , the number of
peaks detected in scalograms and FDWMVSE s among 100 real
CEOAE signals are illustrated in Fig. 2 , where the “ shared ” peaks mean that
these peaks can be detected in both scalograms and FDWMVSEs
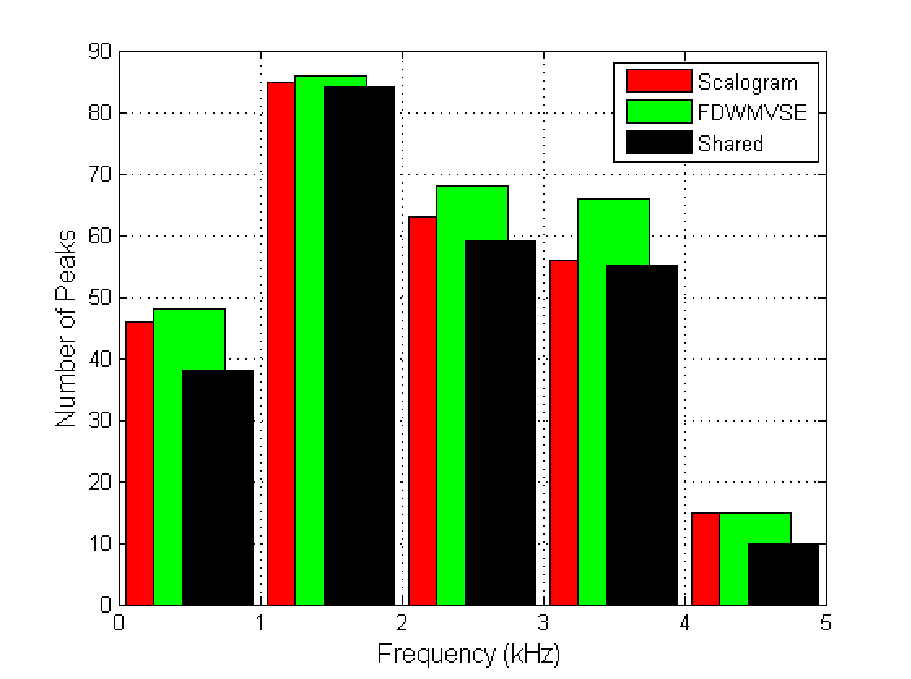
Fig. 2 . Number of peaks
detected in time- frequency representations among 100 real CEOAE
signals using scalogram and
FDWMVSE.
The peak
distributions and the regression lines are illustrated in Fig. 3 . Using the line
identification proposed in [ 7 ] , where the relation of peak latency τ (ms) and peak frequency f (kHz) was expressed
as τ = κ f λ , we can obtain τ = 8.38 f -0.26 for scalogram and τ = 8.45 f -0.28 for FDWMVSE.
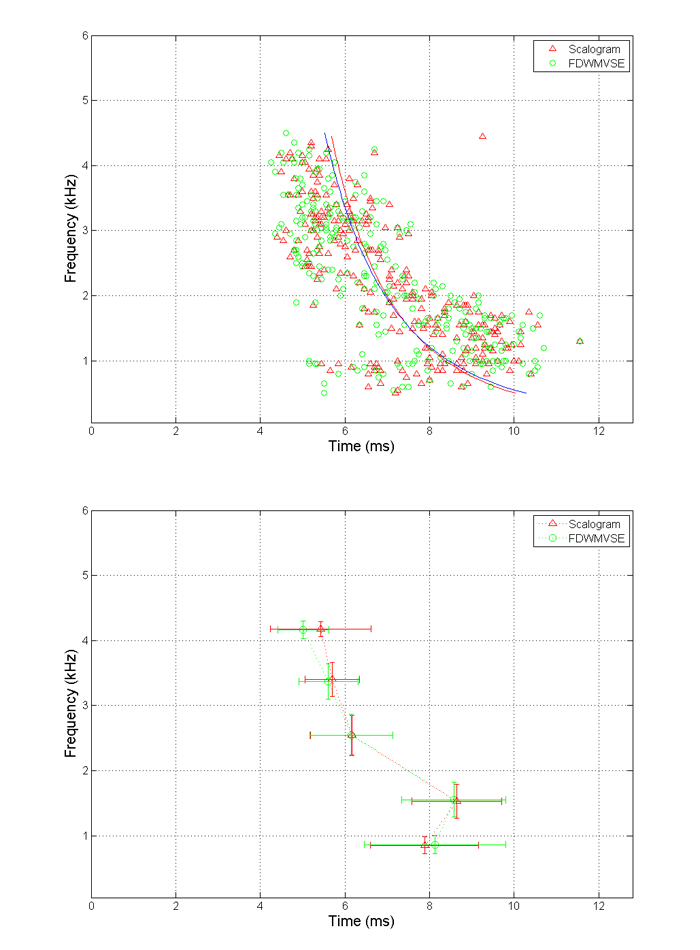
Fig. 3. Distribution of
peaks detected in scalogram and FDWMVSE for 100 real CEOAE
signals : the upper figure
shows the peak
locations and the regression results and the lower figure shows
the mean and standard deviation values of peak locations at
each of five
frequency bands.
Last, the peak width
parameters in time and frequency dimensions using
scalogram and FDWMVSE are shown
and compared in Fig. 4 .
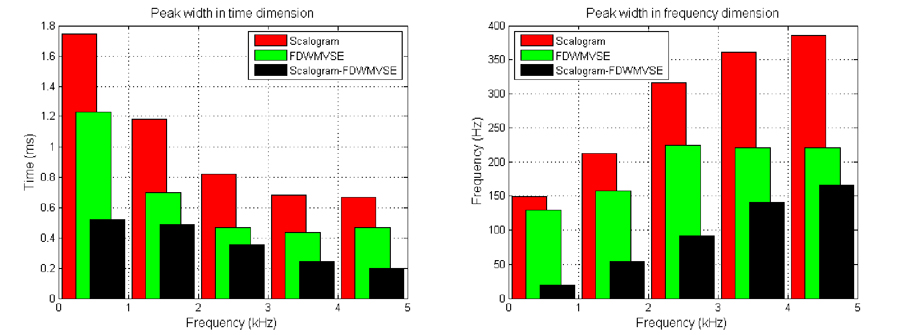
Fig. 4 . P eak width values in time- frequency representations of 100 real CEOAE
signals using scalogram and
FDWMVSE.
4. Discussion
The performances of the FDWMVSE and scalogram were evaluated and
compared using the number of p eaks detected in
the time-frequency distributions among all of the 100 real CEOAE data. It can
be seen from Fig. 2 that, t here were most
peaks in frequency band 1-2 kHz for the two methods , and FDWMVSE reveal ed slightly more
power peaks (more CEOAE components)
especially in frequency band 2 - 4 kHz . The reason may be that the FDWMVSE
method has narrow peak widths in the frequency domain and hence it can distinguish adjacent CEOAE components in
higher frequency bands.
The distributions
of peaks detected by FDWMVSE and by scalogram were
also quite similar in the
time-frequency domain , as seen from Fig.
3 . There were no
significant differences ( p >0.05) for peak
time and peak frequency between the two TFA methods. On the other hand, except
for the peak width in t he
frequency dimension at the frequency band 0 – 1 kHz, all other
peak width values in time and frequency dimensions show significant differences ( p < 0.05) , with the peak
width values of the FDWMVSE method being obviously smaller than those of
scalogram. Fi g.
4 also shows that
the differences of peak width in time dimension between the two methods
decrease with frequency, while the differences in peak width in the frequency
dimension increase with frequency. T he current results
imply that the proposed FDWMVS E
method has good time-frequency
resolution, and this method is expected to have potential applications in CEOAE
studies.
5. Conclusions
The proposed FDWMVSE
method is a good time-frequency analysis method for
CEOAE because it employs
frequency-dependent win dow
sizes to better match the time-frequency characteristics of CEOAEs. By quantitative analysis and
comparison, we can tell that the proposed FDWMVSE method has a similar
performance to the classical wavelet transform in identification of peak locations at each frequency
band. In higher frequency bands, FDWMVSE was able to identify more peaks due to
its high frequency
resolution. The FDWMVSE method can also offer smaller and narrower peak scopes
than the wavelet transform.
Acknowledgement
This study was partially supported
by the University Grants
Council, Hong
Kong, GRF Grant HKU7434/04M.
6. References
[1] Cheng, J., 1995. Time-frequency
analysis of transient evoked otoacoustic emissions via smoothed pseudo Wigner
distribution. Scand . Audiol. 24(2) , 91-96 .
[2] Hatzopoulos , S., Cheng , J. , Grzanka , A. , Martini , A., 2000. Time-frequency
analyses of TEOAE recordings from normal and SNHL patients. Audiology 39(1) , 1-12.
[3] Tognola, G., Grandori, F., Ravazzani , P., 1998. Wavelet analysis of
click-evoked otoacoustic emission. I EEE Trans . Biomed . Eng . 45 (6) , 686 - 697 .
[4] Jedrzejczak, W.
W. , Blinowska, K. J. , Konopka, W. , 2005. Time-frequency
analysis of transiently evoked otoacoustic emissions of subjects exposed to
noise. Hear . Res . 205(1-2) , 249-255 .
[5] Kay, S. M. , 1987. Modern Spectral
Estimation: Theory and Application . Prentice-Hall , Englewood Cliffs,
NJ.
[6] Z hang , Z. G., Zhang, V. W., Chan,
S. C., McPherson, B., Hu, Y., 2008. Time- frequency analysis
of click-evoked otoacoustic emissions by means of a minimum variance spectral
estimation-based method . Hear . Res . 2 43 (1-2) , 18-27 .
[ 7 ] Sisto, R., Morleti,
A., 2002. On the frequency
dependence of the otoacoustic emission latency in hypoac oustic and normal ears . J. Acoust. Soc. Am. 111(1) , 297 - 308.
|